Snow (MET Norway and NR)
Product summary
The main product provided by Sub-service Snow is a fractional snow cover (FSC) product. The product has daily temporal resolution and is provided in pairs covering the two hemispheres. The product is based on a sensor fusion algorithm of AVHRR GAC and SMMR + SSM/I + SSMIS data. The product set consists of a time series starting in 1982 that is extended in batches with data until present. The snow maps are of 5 km spatial resolution with full spatial coverage independent of clouds and polar-night darkness. The product includes an estimate of the uncertainty at grid level.
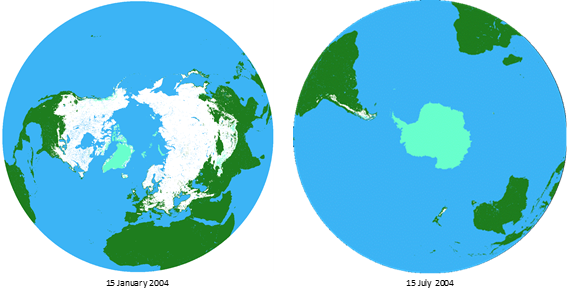
Background
Snow and land ice are key elements of the water cycle in countries at high latitudes and at high elevations. Seasonal snow is characterised by high temporal variability. At a short time scale the extent and properties of the snow cover are driven by meteorological events, so the year-to-year variability is also very high. The variations at daily-to-seasonal time scales are superimposed to long-term trends in the cryosphere. Changes in the snow cover over time are a sensitive indicator of climate change at the global, continental and local scales. The Arctic region is warming faster than the Earth in general, and the corresponding part of the cryosphere is significantly affected. This includes the seasonal snow cover resulting in shorter snow seasons.
Trying to quantify the changes in snow cover regionally and hemispherically, the ESA/NoSA CryoClim project (2008-2013) developed a sub-service for long-term systematic climate monitoring of the snow cover (Solberg et al. 2014). The sub-service was a contribution to the Global Earth Observation System of Systems (GEOSS) and the Global Cryosphere Watch (GCW) following the climate monitoring principles recommended by the Global Climate Observing System (GCOS).
This sub-service was further developed by the ESA/NoSA Sentinel4CryoClim project (2015-2018), which mitigated a number of initial weaknesses with the algorithms and product, included adding uncertainty information and making a much more comprehensive validation (Solberg et al. 2018). An option activity (2020-2022) to the ESA Climate Change Initiative (CCI) Snow ECV project (Snow_CCI) has advanced the multi-sensor multi-temporal algorithm to estimate the fractional snow cover (FSC) (Solberg et al. 2022).
The current time series provides daily snow products of 5 km resolution with global coverage from 1 January 1982 until 30 June 2019. The snow product time series is a unique contribution to the climate community already making significant interest. The time series is one of the longest available, and there are no other such time series of daily and full coverage for both hemispheres. The novel multi-sensor multi-temporal algorithm has made this possible. Alternative time series have either much coarser resolution (due to the use of passive microwave radiometer data only) and are not able to cover the whole Earth on a daily basis (as optical data are limited by cloud cover and the polar night).
Retrieval algorithm
The multi-sensor multi-temporal fusion algorithm is based on a hidden Markov model (HMM) simulating the snow states based on observations with optical sensors and passive microwave radiometers (PMR). The basic idea is to simulate the states the snow surface goes through during the snow season with a state model. The states are not directly observable, but the remote sensing observations give data describing the snow conditions, which are related to the snow states. The HMM solution represents not only a multi-sensor model but also a multi-temporal model. The sequence of states over time is conditioned to follow certain optimisation criteria.
This described approach was originally used to determine the binary snow cover extent (SCE) (Rudjord et al. 2015). In the most recent development, the algorithm was advanced to estimate the fractional snow cover (FSC) down to the 1%-FSC level (Solberg et al. 2022). This is done in two steps. First, more snow states were introduced to be able to estimate the snow cover in 10% categories. Second, we combined a histogram-equalisation approach and interpolation weighted by state probabilities to obtain 1%-FSC precision.
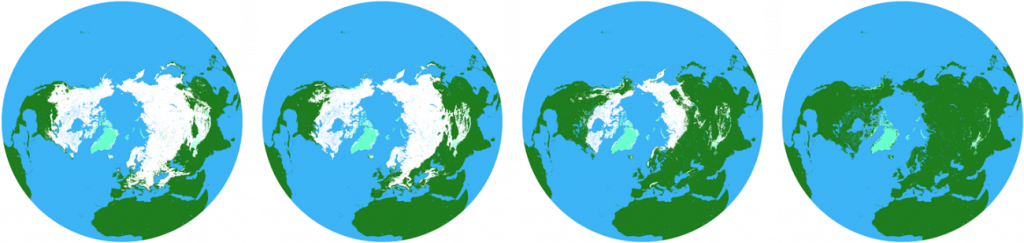
Validation
The validation followed two different approaches. The first was based on using in-situ data following the same approach as was done for the past SCE binary product. The other approach closely followed the ESA Snow ECV (Snow_CCI) project. Based on the in-situ data approach, very similar results to those obtain previously for the binary product, were found. The validation using high-resolution optical data, showed that the overall accuracy is high with an RMSE in the order of 16% and a bias lower than 2.4%. For the FSC uncertainty estimates, a general overestimation of 2-3% was found.
References:
Rudjord, Ø., Salberg, A.-B., Solberg, R., 2015. Global snow cover mapping using a multi-temporal multi-sensor approach. In Proceedings of the 2015 8th International Workshop on the Analysis of Multitemporal Remote Sensing
Solberg, R., Killie, M. Andreassen L. M. and König, M., 2014. CryoClim: A new system and service for climate monitoring of the cryosphere. IOP Conf. Series: Earth and Environmental Science, 17, 012008.
Solberg R., Rudjord Ø., Salberg, A.-B., Killie, M. A., Eastwood, S. and Breivik, L. A., 2018. Advancement of global snow mapping in CryoClim – Sentinel4CryoClim phase 2 report. NR Note SAMBA/44/18, Norwegian Computing Center.
Solberg, R., Rudjord, Ø., Salberg, A.-B., Killie, M. A., Sørensen, A. M., Eastwood, S. (2022) ESA CCI+ Snow ECV, Option 2 – Fractional Snow Cover in CryoClim: Annex to Algorithm Theoretical Basis Document, version 2.0, July 2022.